Chi-Square Test
Groups and Numbers
You research two groups and put them in categories of single, married or divorced:
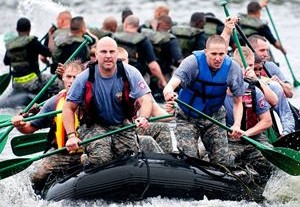
Single: 47 Married: 71 Divorced: 35
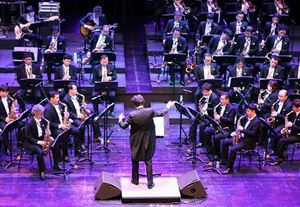
Single: 44 Married: 85 Divorced: 40
The numbers are definitely different, but ...
- Is that just random chance?
- Or have you found something interesting?
The Chi-Square Test gives a "p" value to help you decide!
Example: "Which holiday do you prefer?"
Beach | Cruise | |
Men | 209 | 280 |
Women | 225 | 248 |
Does Gender affect Preferred Holiday?
If Gender (Man or Woman) does affect Preferred Holiday we say they are dependent.
By doing some special calculations (explained later), we come up with a "p" value:
p value is 0.132
Now, p < 0.05 is the usual test for dependence.
In this case p is greater than 0.05, so we believe the variables are independent (ie not linked together).
In other words Men and Women probably do not have a different preference for Beach Holidays or Cruises.
It was just random differences which we expect when collecting data.
Understanding "p" Value
"p" is the probability the variables are independent.
Imagine that the previous example was in fact two random samples of Men each time:
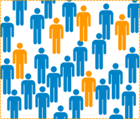
Beach 209, Cruise 280
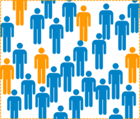
Beach 225, Cruise 248
Is it likely you would get such different results surveying Men each time?
Well the "p" value of 0.132 says that it really could happen every so often.
Surveys are random after all. We expect slightly different results each time, right?
So most people want to see a p value less than 0.05 before they are happy to say the results show the groups have a different response.
Let's see another example:
Example: "Which pet do you prefer?"
Cat | Dog | |
Men | 207 | 282 |
Women | 231 | 242 |
By doing the calculations (shown later), we come up with:
P value is 0.043
In this case p < 0.05, so this result is thought of as being "significant" meaning we think the variables are not independent.
In other words, because 0.043 < 0.05 we think that Gender is linked to Pet Preference (Men and Women have different preferences for Cats and Dogs).
Just out of interest, notice that the numbers in our two examples are similar, but the resulting p-values are very different: 0.132 and 0.043. This shows how sensitive the test is!
Why p<0.05 ?
It is just a choice! Using p<0.05 is common, but we could have chosen p<0.01 to be even more sure that the groups behave differently, or any value really.
Calculating P-Value
So how do we calculate this p-value? We use the Chi-Square Test!
Chi-Square Test
Note: Chi Sounds like "Hi" but with a K, so it sounds like "Ki square"
And Chi is the greek letter Χ, so we can also write it Χ2
Important points before we get started:
- This test only works for categorical data (data in categories), such as Gender {Men, Women} or color {Red, Yellow, Green, Blue} etc, but not numerical data such as height or weight.
- The numbers must be large enough. Each entry must be 5 or more. In our example we have values such as 209, 282, etc, so we are good to go.
Our first step is to state our hypotheses:
Hypothesis: A statement that might be true, which can then be tested.
The two hypotheses are.
- Gender and preference for cats or dogs are independent.
- Gender and preference for cats or dogs are not independent.
Lay the data out in a table:
Cat | Dog | |
Men | 207 | 282 |
Women | 231 | 242 |
Add up rows and columns:
Cat | Dog | ||
Men | 207 | 282 | 489 |
Women | 231 | 242 | 473 |
438 | 524 | 962 |
Calculate "Expected Value" for each entry:
Multiply each row total by each column total and divide by the overall total:
Cat | Dog | ||
Men | 489×438962 | 489×524962 | 489 |
Women | 473×438962 | 473×524962 | 473 |
438 | 524 | 962 |
Which gives us:
Cat | Dog | ||
Men | 222.64 | 266.36 | 489 |
Women | 215.36 | 257.64 | 473 |
438 | 524 | 962 |
Subtract expected from observed, square it, then divide by expected:
In other words, use formula (O−E)2E where
- O = Observed (actual) value
- E = Expected value
Cat | Dog | ||
Men | (207−222.64)2 222.64 | (282−266.36)2 266.36 | 489 |
Women | (231−215.36)2 215.36 | (242−257.64)2 257.64 | 473 |
438 | 524 | 962 |
Which gets us:
Cat | Dog | ||
Men | 1.099 | 0.918 | 489 |
Women | 1.136 | 0.949 | 473 |
438 | 524 | 962 |
Now add up those calculated values:
1.099 + 0.918 + 1.136 + 0.949 = 4.102
Chi-Square is 4.102
From Chi-Square to p
Degrees of Freedom
First we need a "Degree of Freedom"
Degree of Freedom = (rows − 1) × (columns − 1)
For our example we have 2 rows and 2 columns:
DF = (2 − 1)(2 − 1) = 1×1 = 1
p-value
The rest of the calculation is difficult, so either look it up in a table or use the Chi-Square Calculator.
The result is:
p = 0.04283
Done!
Chi-Square Formula
This is the formula for Chi-Square:
Χ2 = Σ(O − E)2E
- Σ means to sum up (see Sigma Notation)
- O = each Observed (actual) value
- E = each Expected value
So we calculate (O−E)2E for each pair of observed and expected values then sum them all up.